Xin-Wei Yao received the Engineering Degree in the College of Mechanical Engineering from the Zhejiang University of Technology (ZJUT), Hangzhou, China, in 2008. Scale network analysis, most of these papers have focused on networks with a strong social component instead of knowl-edge networks. Whether the same characteristics and dynam-ics seen in social networks are applicable to knowledge net-works, however, is still not obvious. Among the limited set of literature that studied knowledge. Binarized neural networks: Training deep neural networks with weights and activations constrained to +1 or −1. ArXiv Preprint arXiv:1602.02830. Google Scholar; M. Duraisamy and F. Mary Magdalene Jane. Cellular neural network based medical image segmentation using artificial bee colony algorithm.
Yu Shi., Xinwei He., Naijing Zhang., Carl Yang, and Jiawei Han. User-Guided Clustering in Heterogeneous Information Networks via Motif-Based Comprehensive Transcription. In Proceedings of the 2019 European Conference on Machine Learning and Principles and Practice of Knowledge Discovery in Databases (ECMLPKDD), 2019. Convolutional neural network Long short-term memory networks Xinwei He received the B.S. Degree in Electronics and Information Engineering from Hebei University of Science and Technology (HEBUST), Shijia zhuang, China in 2013. He is currently a Ph.D. Student in the School of Electronic Information and Communications, HUST.
Download PDFAbstract: Multi-view networks are broadly present in real-world applications. In themeantime, network embedding has emerged as an effective representation learningapproach for networked data. Therefore, we are motivated to study the problemof multi-view network embedding with a focus on the optimization objectivesthat are specific and important in embedding this type of network. In ourpractice of embedding real-world multi-view networks, we explicitly identifytwo such objectives, which we refer to as preservation and collaboration. Thein-depth analysis of these two objectives is discussed throughout this paper.In addition, the mvn2vec algorithms are proposed to (i) study how varied extentof preservation and collaboration can impact embedding learning and (ii)explore the feasibility of achieving better embedding quality by modeling themsimultaneously. With experiments on a series of synthetic datasets, alarge-scale internal Snapchat dataset, and two public datasets, we confirm thevalidity and importance of preservation and collaboration as two objectives formulti-view network embedding. These experiments further demonstrate that betterembedding can be obtained by simultaneously modeling the two objectives, whilenot over-complicating the model or requiring additional supervision. The codeand the processed datasets are available atthis http URL.
Submission history
From: Yu Shi [view email][v1]
Xinwei Networks Network & Wireless Cards Drivers
Fri, 19 Jan 2018 23:14:08 UTC (2,668 KB)[v2] Tue, 30 Oct 2018 20:57:12 UTC (2,668 KB)
[v3]Sun, 3 Nov 2019 01:52:09 UTC (3,031 KB)
Full-text links:
Download:
Current browse context: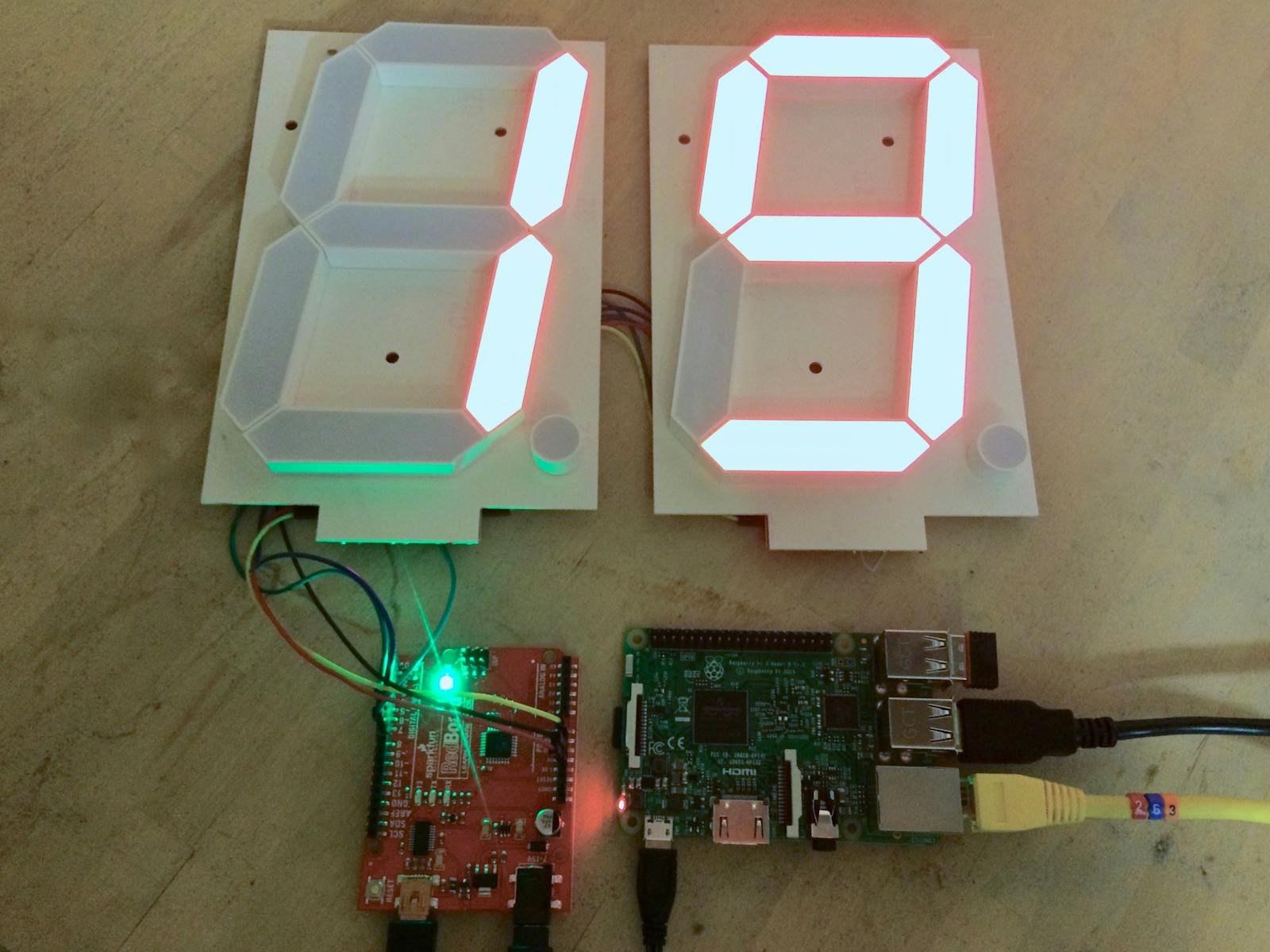
References & Citations
DBLP - CS Bibliography
Fangqiu Han
Xinran He
Carl Yang
Jie Luo

Xinwei Networks Network & Wireless Cards Drivers
arXivLabs is a framework that allows collaborators to develop and share new arXiv features directly on our website.
Both individuals and organizations that work with arXivLabs have embraced and accepted our values of openness, community, excellence, and user data privacy. arXiv is committed to these values and only works with partners that adhere to them.

Have an idea for a project that will add value for arXiv's community? Learn more about arXivLabs and how to get involved.
